Artificial Intelligence And Its Future: What We Should Know In 2023
What is Artificial Intelligence?
The term artificial intelligence (AI) refers to the replication of human intellect in robots programmed to think and act like humans. Machine intelligence may also refer to machines demonstrating human-like characteristics such as learning and problem-solving.
The capacity of artificial intelligence to rationalize and execute actions that have the highest likelihood of reaching a certain objective is its ideal feature.
Machine learning (ML) is a subset of artificial intelligence that refers to the notion that computer systems or neural networks can automatically learn from and adapt to new data without the assistance of humans.
Deep learning approaches facilitate autonomous learning by absorbing massive volumes of either unstructured data or structured data, such as text, photos, or video.
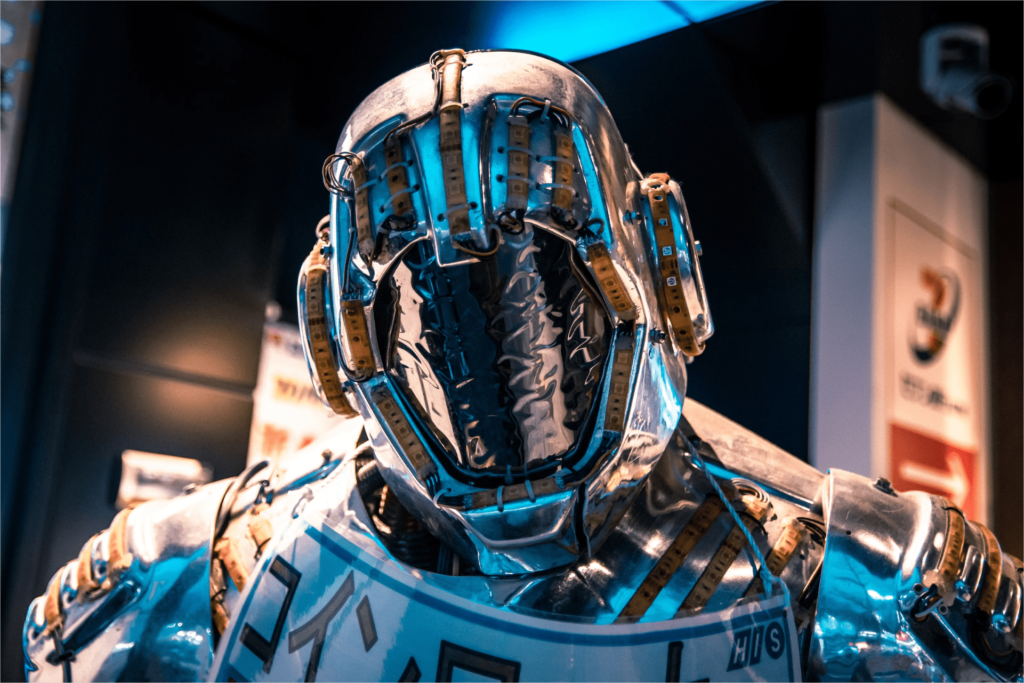
Key Takeaways
- Although there are still several tough obstacles to overcome, artificial intelligence can disrupt several industries.
- A significant problem that requires attention is the need for knowledge and comprehension of AI.
- In AI tasks, human-level precision is challenging and demands significant effort.
- Data security and privacy are crucial when adopting AI, especially when working with delicate data like medical records.
- Numerous industries, including healthcare, banking, and transportation, have adopted AI.
Understanding Artificial Intelligence (AI)
Most people think of robots when they hear the word artificial intelligence. This is because big-budget films, science fiction, and novels weave storylines about human-like robots wreaking havoc on human beings on Earth. However, this could not be further from the truth about human intelligence.
Artificial intelligence is founded on the idea that the human brain and intellect may be characterized so that a machine can easily [duplicate and execute tasks], from the most simple to the most complicated. Artificial intelligence’s aims include imitating the human language and cognitive processes.
To the degree that they can be concretely described, researchers and developers in the field are making quick progress in replicating tasks like learning, reasoning, and perception. Some predict that inventors may soon be able to create systems that outperform humans’ ability to study or reason about any topic.
Others remain doubtful since all cognitive activity is laden with value judgments based on the human experience.
As technology progresses, earlier artificial intelligence criteria become obsolete. Machines that calculate fundamental calculations or detect text using optical character or speech recognition alone, for example, are no longer regarded to possess artificial intelligence since these functions are now assumed to be inherent in computers.
AI is constantly evolving to benefit a wide range of sectors. Machines are wired to humans in natural language processing, utilizing a multidisciplinary method incorporating mathematics, computer science, linguistics, psychology, and other disciplines.
Types of Artificial Intelligence
Artificial intelligence is classified into two types: weak and powerful.
Weak artificial intelligence software is a system built to do a single task. Weak AI systems include video games like the chess example mentioned before and even personal assistants or virtual assistants of personal assistants, like Amazon’s Alexa and Apple’s Siri. You ask the weak ai- programs assistant a question, and it responds.
Strong artificial intelligence systems do not always require human intervention for intelligence-like functions. These are often more sophisticated and difficult systems. They are trained to handle circumstances in which they may be required to solve problems without human intervention or of a fully human mind. These systems are used in applications like self-driving cars re-driving automobiles, and hospital operating rooms.
What Are the 4 Types of AI?
Artificial intelligence can be categorized into one of the ai examples of four types.
- Reactive AI employs algorithms to optimize outputs in response to a collection of inputs. For example, chess AIs’ are reactive systems that optimize the optimal strategy to win the game. Reactive AI is typically static, incapable of learning or adapting to unexpected conditions. As a result, given equal inputs, it will create the same output.
- Limited Memory AI may adapt to previous experience or update itself in response to new observations or data. The updating quantity is frequently limited (hence the term), and memory is small. Self-driving cars, for example, can “read the road” and adjust to new conditions, even “learning” from previous experience.
- Theory-of-mind AI is adaptable and has a strong ability to learn and remember previous experiences. Advanced chatbots that can pass the Turing Test can deceive a person into thinking the AI is a human. These AI, while smart and amazing, are not self-aware.
- Self-aware AI becomes conscious and aware of its existence. Some scientists feel that an AI will never become aware or “alive” since it is still in the realm of science fiction.
A Brief History of Artificial Intelligence
Here’s a quick look at examples of how artificial intelligence has grown over the last six decades.
1956 – John McCarthy created the phrase ‘artificial intelligence’ and hosted the first AI conference.
1969 – Shakey was the first general-purpose mobile robot constructed. It can now accomplish things with a purpose rather than merely a set of instructions.
1997 – The supercomputer ‘Deep Blue’ defeated the world champion chess player in a match. IBM’s creation of the ai program for this gigantic computer was a huge step forward.
2002 – Development of the first commercially effective robotic vacuum cleaner.
2005 – 2019 – Speech recognition, artificial general intelligence, robotic process automation (RPA), dancing robot, self-driving cars, smart houses, and more innovations are among ai technologies debuting today.
2020 – During the early phases of the SARS-CoV-2 (COVID-19) epidemic, Baidu makes the LinearFold AI algorithm available to medical and scientific organizations working on a vaccine. The same computer vision program predicts the virus’s RNA sequence in under 27 seconds, 120 times quicker than prior approaches.
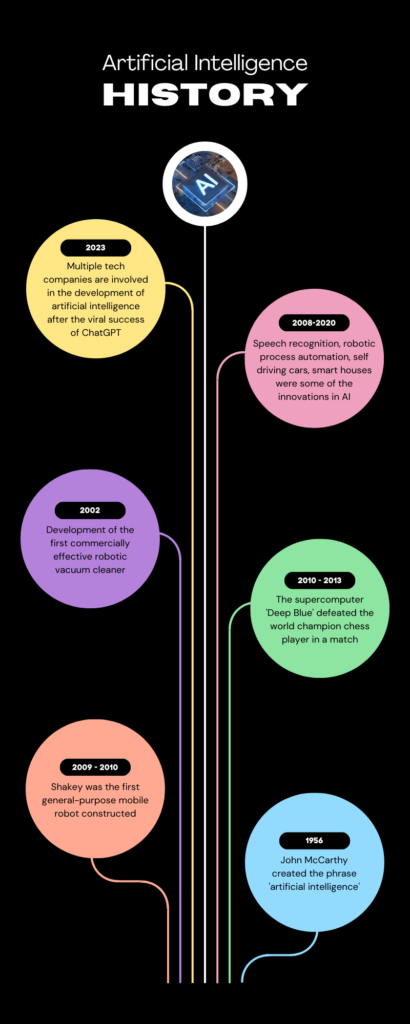
Top Common Challenges in AI
1. Computing Capability
Most developers are turned off by how much power these power-hungry supervised learning algorithms use. Machine Learning and Deep Learning are the building blocks of modern Artificial Intelligence, requiring an increasing number of cores and GPUs to function properly.
We have concepts, ai projects, ai tools, and ai technologies, techniques, and skills to apply deep learning frameworks in ai applications in various fields such as asteroid tracking, healthcare deployment, cosmic body tracing, and much more.
They necessitate the computational capacity of a supercomputer, yet supercomputers are not cheap. Although the availability of Cloud Computing and parallel processing systems allow engineers to work more successfully on AI systems, it comes with a cost.
With a growth in the intake of enormous amounts of data and quickly expanding complicated algorithms, not everyone can afford that.
2. Lack of Trust
One of the most concerning aspects of AI is the uncertain nature of how deep learning models forecast the output of ai algorithms or techniques. Understanding how a machine using deep learning techniques model certain collection of inputs might design a solution for ai techniques or algorithms for many issues takes time for narrow ai or layperson.
Many people worldwide need to be made aware of the usage or presence of artificial intelligence limited memory ai important or using artificial intelligence strong ai important general intelligence and how it is interwoven into common objects they interact with, such as smartphones, smart TVs, banking, and even automobiles and autonomous vehicles (at some level with strong ai and strong ai with capabilities of automation).
3. Lack of Knowledge
Although there are many areas in the industry where Artificial Intelligence may be used as a narrow ai superior alternative to traditional methods, the fundamental issue is with ai research, ai solutions themselves, and the need for more understanding of Artificial Intelligence. Apart from early ai research from technology enthusiasts, college students, and academics, only with machine learning ai research, and few individuals know AI’s potential.
Many SMEs (Small and Medium Enterprises), for example, can have their work scheduled or learn innovative ways to increase production, manage resources, sell and manage products online, learn and understand consumer behaviour, and respond to the market effectively and efficiently. They need to be made aware of service providers in the IT business, such as Google Cloud, Amazon Web Services, and others.
4. Human-level
This is one of the most critical issues in AI, and it has kept academics on the lookout for AI services in businesses and start-ups. These firms may claim to exceed 90% accuracy, yet people can outperform them in these circumstances.
Let our first computer program and computer vision and model predict whether the image is of a dog or a cat, for example. Humans can almost always predict the proper outcome, with an astounding accuracy of more than 99%.
A deep learning model would need advanced ai algorithms, unparalleled fine-tuning, hyperparameter optimization, a big dataset, a well-defined and accurate algorithm, resilient computational capacity, continuous training on train data, and testing to achieve comparable results on test data. That sounds like a lot of effort and is a hundred times more difficult than it appears.
Using a service provider to train particular deep learning models using pre-trained models is one approach to avoid performing all of the hard work. They’ve been trained on millions of photos and fine-tuned for optimum accuracy, but the fundamental issue with deep neural networks is that they still need to make mistakes and would struggle to match human performance.
5. Data Security and Privacy
The availability of data and resources to train deep and machine learning models is the primary factor on which all deep and machine learning models are based. Yes, we have data, but because this data is created by millions of people throughout the world, there is a risk that it may be misused.
Assume a medical service provider provides services to one million individuals in a city. A cyber-attack results in the personal data of all one million customers falling into the hands of anybody on the dark web.
This information contains illnesses, health issues, medical history, etc. To make matters worse, we are now dealing with data on the size of planets. With this new data coming in from all angles, there would likely be some data leakage.
Some businesses have already begun to work creatively to overcome these obstacles. Because it trains the data on smart devices, it is not transported back to the servers; only the trained model is returned to the company.
6. The Bias Issue
The quality of an AI system is determined by the amount of data on which it is trained. As a result, the capacity to obtain excellent training data is the future solution to good AI systems. However, the everyday data organizations collect labelled training data needs to be stronger and more meaningful.
They are prejudiced and only describe the character and specifications of a human being or a small group of individuals with common interests based on religion, ethnicity, gender, community, and other racial prejudices.
Only by establishing a strong neural network with enough ai tools and programs and certain AI tools and algorithms that can efficiently track these challenges can genuine change be brought about.
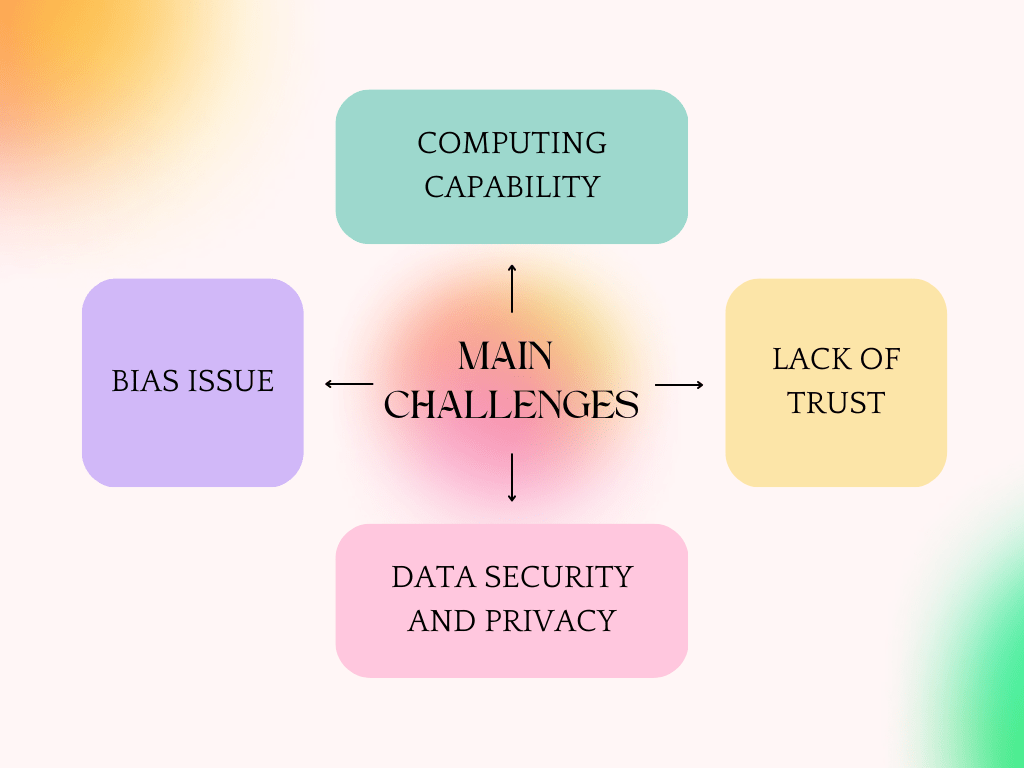
What is Machine Learning?
Artificial intelligence (AI) is a subset that includes machine learning, which allows computers to learn and make predictions or decisions based on data inputs.
Simply put, it is the computer science part of teaching computers to learn and behave like people without explicit programming. Machine learning relies on statistical algorithms that improve with experience and more data exposure.
Importance of Machine Learning
In today’s data-driven environment, machine learning is crucial. It helps decision-makers improve efficiency and cut costs across various industries, including healthcare, banking, marketing, and transportation.
Massive volumes of data are analyzed using machine learning algorithms to find patterns people cannot see. These algorithms can then be applied to process optimization, anomaly detection, and outcome prediction.
Types of Machine Learning
Machine learning algorithms typically perform tasks commonly fall into one of three categories:
Supervised learning
The most prevalent kind of a machine learning model is supervised learning. A model must be trained using labelled data to predict brand-new, unforeseen data.
The input variables (features) describe data, and the output variables (labels) comprise the labelled data. The model produces predictions on new data after learning from the labelled data.
Unsupervised learning
Training a model on unlabeled data to find patterns and relationships is known as unsupervised learning. Without any supervision or instruction, the model learns from the data. Clustering, anomaly detection, and dimensionality reduction can benefit from unsupervised learning.
Reinforcement Learning
Through reinforcement learning, a model is taught to make choices depending on input from the outside world. The model makes decisions to maximize rewards and reduce penalties after learning through trial and error.
What is Deep Learning?
Artificial neural network or networks are used in deep learning, a branch of machine learning, to model and resolve complicated issues. These neural networks, which have numerous layers of connected nodes, were designed to mimic the structure and operation of the human brain.
Deep learning algorithms can autonomously learn hierarchical and structured data and representations, which enables them to recognize intricate patterns and connections.
How Deep Learning Works
Deep learning algorithms function by picking the best representations of the input data at various levels. The neural network’s initial layer handles the unprocessed input data, and each successive layer processes the output of the one before it.
The model can learn more abstract representations of the data as each layer extracts increasingly complicated aspects from the input data.
Backpropagation is a technique for training deep learning algorithms that includes altering the neural network weights in response to the difference between the predicted and actual results. This technique has multiple iterations until the model’s performance is adequate.
Applications of Deep Learning
State-of-the-art performance in various tasks, including picture and speech recognition, natural language processing, and game playing, has been attained using deep learning.
It has also been applied in ai applications in scientific disciplines like bioinformatics and drug discovery to evaluate complex data and produce predictions.
Future of Deep Learning
Deep learning is quickly developing, with new developments and discoveries being made every year. Researchers are developing new algorithms and architectures to learn from even bigger and more detailed information.
Deep learning can transform many industries and address some of the most difficult problems in the world. Thus the future of this technology appears bright.
Deep learning is a branch of machine learning that model and resolves complicated issues using artificial neural networks. It operates by teaching different levels of representations of the input data, and it has been applied to accomplish cutting-edge performance on several tasks.
Deep learning can transform many industries and address some of the most difficult problems in the world. Thus the future of this technology appears bright.
Human Intelligence vs Artificial Intelligence
Human intelligence is the capacity for knowledge, understanding, and problem-solving. It is a complicated idea with several facets encompassing many cognitive abilities like perception, attention, memory, and decision-making. Creativity, emotional intelligence, and social skills—which enable us to build connections and communicate effectively—are other traits of human intelligence.
The ability of robots to carry out activities that ordinarily need human intellect, such as speech recognition, decision-making, and language translation, is known as artificial intelligence, on the other hand. Data and algorithms, which allow machines to learn and improve over time, are the foundation of artificial intelligence.
Human intellect continues to be considerably superior to AI in several skills, including complex problem-solving, pattern recognition, and creativity. Humans can think abstractly, connect seemingly unconnected concepts, and develop original solutions to challenging issues.
AI, however, excels at jobs that call for quickness, precision, and consistency. Machines can quickly process enormous amounts of data, spot patterns people would miss, and reach conclusions using unbiased criteria. Additionally, AI is not subject to human prejudices or emotions, occasionally impairing our judgment.
Machines will become better at performing tasks that were formerly assumed to be reserved for people as AI develops. This does not imply that machines will entirely replace people, though. Instead, a collaboration between people and machines that complements each other’s strengths and shortcomings is more likely to be the model for intelligence in the future.
In conclusion, natural and artificial intelligence are useful and required for various activities. Artificial intelligence important as general intelligence (AI) is best suited for activities that call for speed, accuracy, and consistency, whereas human intelligence excels at the creativity and sophisticated problem-solving. The distinction between the human mind and artificial general intelligence will become hazier as technology develops. Still, it is crucial to remember that each has particular advantages and disadvantages that should be taken advantage of to the fullest extent possible.
How AI Can Improve Businesses In the Coming Years

Large corporations like Apple and Google have significantly invested in developing artificial intelligence technology. Aside from those industries, AI is routinely underutilized in others, such as manufacturing, education, retail, and healthcare.
Every day, these organizations generate vast amounts of data, but AI is rarely utilized to analyze massive datasets and make inferences from the patterns and characteristics of that data.
The important question is, why is this such narrow a big deal? The cause is that weak ai technologies cannot yet regulate ai’ strong need for more access, comprehension, and ability. We’ve read many ai researchers’ research about the major AI issues. We must use technology to determine what can bridge the gap between AI challenges and commercial profitability.
One of the most serious artificial intelligence issues is that most enterprises need more sophisticated and expensive processing resources. Furthermore, they need access to the expensive and rare AI knowledge necessary to efficiently employ such resources.
The global AI market value AI capabilities in their work. 37% of firms had previously used or planned to use AI services; according to one estimate, the AI business will be worth $126 billion annually by 2025.
According to Forbes, AI will be worth $15.7 trillion by 2030, with investments reaching $500 billion by 2024.
Use Cases of Artificial Intelligence in Multiple Industries
- Healthcare: Medical imaging is one of the primary areas where AI significantly influences healthcare. Image recognition algorithms driven by AI can examine X-rays, CT scans, and MRIs to find anomalies that human radiologists may miss. This can result in faster and more accurate diagnoses, eventually saving lives.
- Finance: Another sector where AI is widely employed is fraud detection. The algorithms can examine transaction patterns for abnormalities suggesting fraudulent behaviour. This can assist banks and financial organizations avoid financial losses due to fraud.
- Retail: AI is used by online merchants such as Amazon and Netflix to customize product suggestions based on user behaviour and preferences. These organizations can recommend items and services more likely to appeal to specific clients by evaluating purchase history, browsing behaviour, and other data.
- Manufacturing: AI is assisting manufacturers in reducing downtime and increasing production efficiency through predictive maintenance. By evaluating data from sensors and other sources, machine learning algorithms can forecast when equipment will likely fail and proactively plan maintenance before a breakdown.
- Transportation: One of the most apparent uses of AI in transportation is autonomous cars. Self-driving vehicles and trucks have the potential to be safer and more efficient than human drivers, thereby lowering accidents and increasing traffic flow.
- Education: Another area where AI significantly influences is adaptive learning systems. These systems may customize learning experiences for individual students by assessing student performance data and offering them specific feedback and ideas for development.
- Agriculture: AI is used in precision agriculture to maximize crop productivity and eliminate waste. Machine learning algorithms can help farmers decide when to sow, water, and harvest crops by evaluating data from sensors, drones, and other sources.
- Energy: AI is assisting utilities in better managing energy distribution and use through smart grid management. Machine learning algorithms can estimate energy consumption and regulate supply accordingly by evaluating data from sensors and other sources, decreasing waste, and enhancing efficiency.
- Gaming: AI-powered gaming avatars are getting more popular, particularly in multiplayer games. These characters may adapt to the player’s actions and preferences, making the game more interesting and difficult.
- Marketing: AI is used in predictive analytics to forecast customer behaviour and optimize marketing strategies. Machine learning algorithms may find patterns and trends in data from many sources, informing decisions regarding product development, pricing, and advertising.
- Insurance: AI is being utilized in risk assessment to examine data from diverse sources to predict the possibility of insurance claims. This can assist insurers in identifying high-risk policies and appropriately pricing them.
- Legal: AI-powered natural language processing (NLP) algorithms are being utilized to automate traditionally time-consuming and labour-intensive document analysis and contract assessment operations. These algorithms can recognize essential phrases and clauses in enormous volumes of text-based data, identifying regions that deserve more attention.
- Human resources: AI is utilized to evaluate and analyze job candidates in recruitment and hiring. Machine learning algorithms can identify the individuals most likely to be a good fit for a specific post by examining resumes, cover letters, and other data.
- Real estate: AI-powered algorithms are being utilized to evaluate the worth of homes and other properties in automated property valuation. These algorithms can generate more accurate appraisals than traditional techniques by examining data from many sources, including recent sales and evaluations.
- Hotel: Chatbots and voice assistants are becoming more widespread in the hotel business. Customers may use these tools to learn about services and facilities and receive customized suggestions based on their interests.
- E-commerce: AI-powered chatbots are utilized for customer care and sales help in e-commerce. These chatbots can answer queries about products and services and propose new items that may be of interest by evaluating client data.
- Entertainment: Content recommendation engines utilize AI to propose movies, TV programs, and music based on user tastes. By assessing watching and listening habits, machine learning algorithms can propose material more likely to appeal to specific users.
- Construction: AI enhances safety and quality assurance on construction sites. Machine learning algorithms can identify possible risks and assist in avoiding accidents by evaluating data from sensors and other sources.
- Government: AI is used in predictive policing to detect crime areas and deliver resources accordingly. Machine learning algorithms can find patterns and trends in crime data, informing judgments on where to devote law enforcement resources.
- Sports: AI-powered sensors and data analytics guide training and coaching decisions in athlete performance monitoring. By processing data from embedded sensors
AI War: Google vs Microsoft

Microsoft and Google are ready to square off in a war for the future of search. Microsoft intends to integrate OpenAI’s ChatGPT chatbot into the Bing search engine. Google has already attempted to foreshadow the news by making a hasty announcement [to unveil Bard], its competitor to ChatGPT, and promising further information on its AI future.
The developments placed the two tech behemoths, notorious for previous clashes, on a collision path as they strive to define the next generation of search.
Both businesses are vying for a revolutionary new future for search engines, one in which the results resemble short, clear answers created by AI rather than a collection of links and boxes to click on.
Yesterday, Google teased their Bard chatbot with inquiries similar to OpenAI’s ChatGPT. And today, Microsoft is anticipated to expand its Bing search goals by adding a ChatGPT-like interface that will answer queries in a manner that no search engine has before.
The more human-like responses might revolutionize search. Last year, OpenAI’s ChatGPT brought conversational AI to the mainstream, and if the Bing integration works as intended, the use cases can genuinely shave hours off of research, spreadsheets, coding, and much more).
Microsoft might have an advantage over ChatGPT as we know it now. While ChatGPT is based on GPT-3.5, a big language model published last year, Bing’s chat feature is based on the yet-to-be-announced GPT-4 model. The AI community is still debating how strong GPT-4 will be.
Google has also invested $300 million in Anthropic, an AI business formed by former OpenAI researchers.
Anthropic researches AI and natural language processing models and have created Claude, a competitor to ChatGPT. Anthropic’s chatbot has yet to be available to the public. Still, the company recently announced that Google Cloud is its “preferred cloud provider,” similar to how Microsoft is OpenAI’s exclusive cloud provider thanks to a multibillion-dollar investment.
Summary
Artificial intelligence is a rapidly expanding field with enormous commercial improvement potential. Businesses that use AI to their full potential can reduce costs, boost productivity, and outperform their competitors.
But applying AI has its challenges. Before AI realizes its full potential, several obstacles must be resolved, including a lack of comprehension, data security, prejudice, and performance on par with humans.
Lack of awareness of AI’s potential is one of its biggest problems. Many small and medium-sized businesses (SMEs) are unaware of AI’s capabilities, even though tech enthusiasts, college students, and academics are.
AI has several SME applications, including scheduling tasks, boosting output, managing resources labeled training data, and deciphering consumer behaviour. To overcome this obstacle, AI service providers must promote their offerings and inform businesses of AI’s advantages.
Regarding AI, data security and privacy are also major issues. The data must be protected from misuse because deep learning and machine learning require enormous datasets to build their models.
In a cyberattack, private information may be taken, endangering people’s privacy. One solution to this problem is to train models on smart devices to get around having to send data back to servers. Only the trained model is returned to the business, guaranteeing the security of sensitive information.
Another issue that needs to be addressed is biased. The volume of data used to train an AI system affects how well it performs. It is crucial to collect great data to prevent bias in AI systems.
Based on racial stereotypes towards people of a particular religion, race, gender, or community, AI systems may be biased and only depict a few people who share those preferences. Real change can be achieved by establishing specific algorithms that effectively track these difficulties.
One of the most important problems in AI is performing at the level of humans. Although some businesses assert that their accuracy exceeds 90%, consumers can frequently outperform them.
Deep learning models require unmatched fine-tuning, hyperparameter optimization, a large dataset, a precise and well-defined method, robust computational capability, continuous training on train data, and test data testing to produce similar results. It isn’t easy to match human performance modern neural networks because of this.
Using a service provider to train specific deep learning models using pre-trained models is one approach to avoid doing all the labour-intensive work yourself. After being trained on millions of pictures, these models have been optimized for maximum accuracy. They would have difficulty performing as well as people because they still need to make mistakes.
Despite these difficulties, AI is revolutionizing numerous industries. AI increases efficiency, reduces costs, and makes data-driven decisions in various industries, including healthcare, banking, retail, and agriculture.
To help individuals make better decisions, machine learning algorithms can examine enormous data volumes and uncover patterns humans cannot notice. Deep learning, a subfield of machine learning, is used to model and solve complicated problems. It has been used to analyze complex data and make predictions in scientific fields, including bioinformatics and drug discovery.
AI does not take the place of human intelligence, to sum up. Human intelligence excels at the creativity and complex problem-solving, while artificial intelligence is best suited for tasks that need speed, accuracy, and consistency.
Human and artificial intelligence are complementary in their strengths and weaknesses. The line between human and artificial intelligence will blur as technology advances, so it’s critical to remember that each has unique benefits and drawbacks that should be utilized to the best extent possible.
In conclusion, AI has enormous promise and is already changing many different industries. Despite AI’s difficulties, investing in AI is worthwhile to get an advantage over competitors. Artificial intelligence (AI) cannot and should not replace human intelligence.
Nevertheless, it can support human intellect and assist decision-makers in reaching more informed choices. Companies may reduce costs, increase productivity, and maintain an advantage over rivals by investing in AI and addressing the issues it faces.
FAQ
What is artificial intelligence?
Artificial intelligence (AI) is a branch of computer science that focuses on building robots to learn and carry out tasks that traditionally require human intelligence, such as interpreting natural language, speech recognition, and decision-making.
What are the challenges of implementing AI in businesses?
Lack of understanding, data security and privacy concerns, human-level accuracy, and bias are some difficulties associated with integrating AI in enterprises.
What is machine learning?
A subset of artificial intelligence called “machine learning” enables computers to learn and make predictions or judgments based on data inputs. It is based on statistical algorithms that improve with practice and more data exposure.
What are the types of machine learning?
Supervised learning, unsupervised learning, and reinforcement learning are the three categories of machine learning.
What is deep learning?
Artificial neural networks are used in the deep learning subfield of machine learning to model and solve complex problems. These neural networks, which consist of many layers of interconnected nodes, were created to resemble the organization and function of the human brain.
How can AI improve businesses in the coming years?
By boosting productivity and reducing costs across a range of sectors, including healthcare, finance, retail, and transportation, AI can boost organizations in the years to come. Predictive analytics is another application of AI that can be used to foresee consumer behaviour and improve marketing plans.